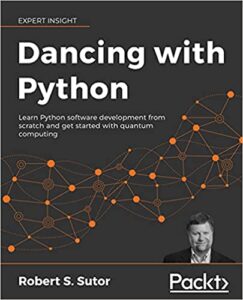
My new book Dancing with Python: Learn Python software development from scratch and get started with quantum computing is now available for purchase from Amazon and Packt Publishing.
Develop skills in Python by implementing exciting algorithms, including mathematical functions, classical searching, data analysis, plotting data, machine learning techniques, and quantum circuits.
Key Features
Learn Python basics to write elegant and efficient code
Create quantum circuits and algorithms using Qiskit and run them on quantum computing hardware and simulators
Delve into Python’s advanced features, including machine learning, analyzing data, and searching
Contributors
About the author
About the reviewer
Contents
List of Figures
Preface
Why did I write this book?
For whom did I write this book?
What does this book cover?
What conventions do I use in this book?
Get in touch
1 Doing the Things That Coders Do
1.1 Data
1.2 Expressions
1.3 Functions
1.4 Libraries
1.5 Collections
1.6 Conditional processing
1.7 Loops
1.8 Exceptions
1.9 Records
10 Contents
1.10 Objects and classes
1.11 Qubits
1.12 Circuits
1.13 Summary
I Getting to Know Python
2 Working with Expressions
2.1 Numbers
2.2 Strings
2.3 Lists
2.4 Variables and assignment
2.5 True and False
2.6 Arithmetic
2.7 String operations
2.8 List operations
2.9 Printing
2.10 Conditionals
2.11 Loops
2.12 Functions
2.13 Summary
3 Collecting Things Together
3.1 The big three
3.2 Lists
3.3 The joy of O(1)
3.4 Tuples
3.5 Comprehensions
3.6 What does “Pythonic” mean?
3.7 Nested comprehensions
3.8 Parallel traverse
3.9 Dictionaries
3.10 Sets
3.11 Summary
4 Stringing You Along
4.1 Single, double, and triple quotes
4.2 Testing for substrings
4.3 Accessing characters
4.4 Creating strings
4.5 Strings and iterations
4.6 Strings and slicing
4.7 String tests
4.8 Splitting and stripping
4.9 Summary
5 Computing and Calculating
5.1 Using Python modules
5.2 Integers
5.3 Floating-point numbers
5.4 Rational numbers
5.5 Complex numbers
5.6 Symbolic computation
5.7 Random numbers
5.8 Quantum randomness
5.9 Summary
6 Defining and Using Functions
6.1 The basic form
6.2 Parameters and arguments
6.3 Naming conventions
6.4 Return values
6.5 Keyword arguments
6.6 Default argument values
6.7 Formatting conventions
6.8 Nested functions
6.9 Variable scope
6.10 Functions are objects
6.11 Anonymous functions
6.12 Recursion
6.13 Summary
7 Organizing Objects into Classes
7.1 Objects
7.2 Classes, methods, and variables
7.3 Object representation
7.4 Magic methods
7.5 Attributes and properties
7.6 Naming conventions and encapsulation
7.7 Commenting Python code
7.8 Documenting Python code
7.9 Enumerations
7.10 More polynomial magic
7.11 Class variables
7.12 Class and static methods
7.13 Inheritance
7.14 Iterators
7.15 Generators
7.16 Objects in collections
7.17 Creating modules
7.18 Summary
8 Working with Files
8.1 Paths and the file system
8.2 Moving around the file system
8.3 Creating and removing directories
8.4 Lists of files and folders
8.5 Names and locations
8.6 Types of files
8.7 Reading and writing files
8.8 Saving and restoring data
8.9 Summary
II Algorithms and Circuits
9 Understanding Gates and Circuits
9.1 The software stack
9.2 Boolean operations and bit logic gates
9.3 Logic circuits
9.4 Simplifying bit expressions
9.5 Universality for bit gates
9.6 Quantum gates and operations
9.7 Quantum circuits
9.8 Universality for quantum gates
9.9 Summary
10 Optimizing and Testing Your Code
10.1 Testing your code
10.2 Timing how long your code takes to run
10.3 Optimizing your code
10.4 Looking for orphan code
10.5 Defining and using decorators
10.6 Summary
11 Searching for the Quantum Improvement
11.1 Classical searching
11.2 Quantum searching via Grover
11.3 Oracles
11.4 Inversion about the mean
11.5 Amplitude amplification
11.6 Searching over two qubits
11.7 Summary
III Advanced Features and Libraries
12 Searching and Changing Text
12.1 Core string search and replace methods
12.2 Regular expressions
12.3 Introduction to Natural Language Processing
12.4 Summary
13 Creating Plots and Charts
13.1 Function plots
13.2 Bar charts
13.3 Histograms
13.4 Pie charts
13.5 Scatter plots
13.6 Moving to three dimensions
13.7 Summary
14 Analyzing Data
14.1 Statistics
14.2 Cats and commas
14.3 pandas DataFrames
14.4 Data cleaning
14.5 Statistics with pandas
14.6 Converting categorical data
14.7 Cats by gender in each locality
14.8 Are all tortoiseshell cats female?
14.9 Cats in trees and circles
14.10 Summary
15 Learning, Briefly
15.1 What is machine learning?
15.2 Cats again
15.3 Feature scaling
15.4 Feature selection and reduction
15.5 Clustering
15.6 Classification
15.7 Linear regression
15.8 Concepts of neural networks
15.9 Quantum machine learning
15.10 Summary
Appendices
A Tools
A.1 The operating system command line
A.2 Installing Python
A.3 Installing Python modules and packages
A.4 Installing a virtual environment
A.5 Installing the Python packages used in this book
A.6 The Python interpreter
A.7 IDLE
A.8 Visual Studio Code
A.9 Jupyter notebooks
A.10 Installing and setting up Qiskit
A.11 The IBM Quantum Composer and Lab
A.12 Linting
B Staying Current
B.1 python.org
B.2 qiskit.org
B.3 Python expert sites
B.4 Asking questions and getting answers
C The Complete UniPoly Class
D The Complete Guitar Class Hierarchy
E Notices
E.1 Photos, images, and diagrams
E.2 Data
E.3 Trademarks
E.4 Python 3 license
F Production Notes
References
Other Books You May Enjoy
Index
Index Formatting Examples
Python function, method, and property index
Python class index
Python module and package index
General index
Share this:
- Threads
-
Categories Dancing with Python, Quantum Computing Tags algorithm, Amazon, coding, Dancing with Python, IBM, machine learning, mathematics, Packt, Python, Qiskit, quantum computing, qubit
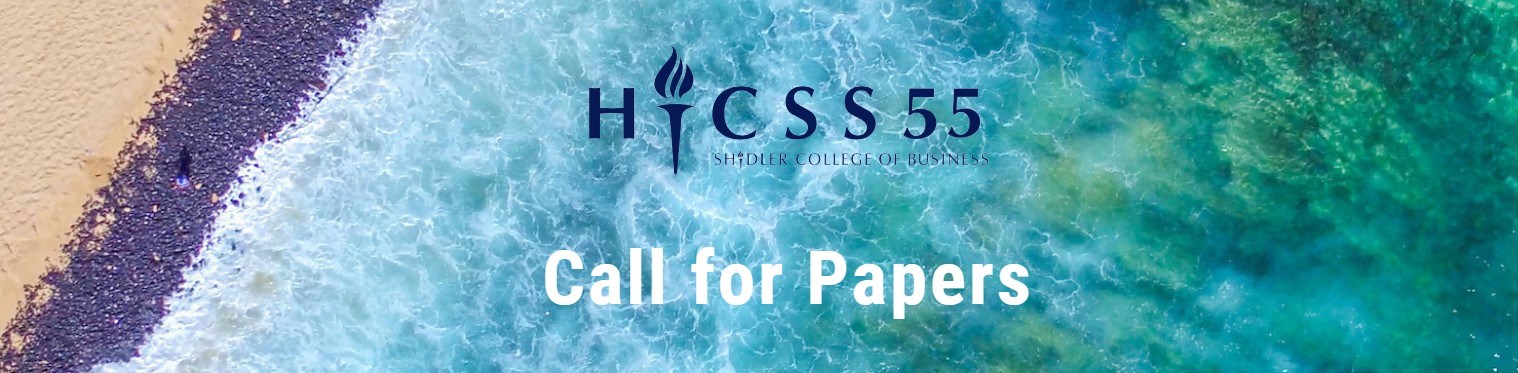
My IBM Quantum colleague Dr. Andrew Wack and I are hosting a minitrack at the Hawaii International Conference on System Sciences (HICSS) 2022.
The description of the minitrack is:
There is no question that quantum computing will be a technology that will spur breakthroughs in natural science, AI, and computational algorithms such as those used in finance. IBM, Google, Honeywell, and several startups are working hard to create the next generation of “supercomputers” based on universal quantum technology.
What exactly is quantum computing, how does it work, how do we teach it, how do we leverage it in education and research, and what will it take to achieve these quantum breakthroughs?
The purpose of this minitrack is to bring together educators and researchers who are working to bring quantum computing into the mainstream.
We are looking for reports that
- improve our understanding of how to integrate quantum computing into business, machine learning, computer science, and applied mathematics university curriculums,
- describe hands-on student experiences with the open-source Qiskit quantum software development kit, and
- extend computational techniques for business, finance, and economics from classical to quantum systems.
It is part of the Decision Analytics and Service Science track at HICSS.
Please consider submitting a report and sharing this Call for Papers with your colleagues.
Share this:
- Threads
-
Categories Quantum Computing Tags AI, algorithm, analytics, computer science, finance, Google, IBM, machine learning, mathematics, Qiskit, quantum computing, Universal Quantum
In section 1.5 of my quantum computing book Dancing with Qubits, I discuss potential applications of the technology to financial services. An excellent survey article by my IBM Quantum colleagues is now on arXiv that updates and goes into much greater detail than what I covered.
“Quantum computing for Finance: state of the art and future prospects” by Daniel J. Egger, Claudio Gambella, Jakub Marecek, Scott McFaddin, Martin Mevissen, Rudy Raymond, Andrea Simonetto, Stefan Woerner, and Elena Yndurain has this abstract:
This paper outlines our point of view regarding the applicability, state of the art, and potential of quantum computing for problems in finance. We provide an introduction to quantum computing as well as a survey on problem classes in finance that are computationally challenging classically and for which quantum computing algorithms are promising. In the main part, we describe in detail quantum algorithms for specific applications arising in financial services, such as those involving simulation, optimization, and machine learning problems. In addition, we include demonstrations of quantum algorithms on IBM Quantum back-ends and discuss the potential benefits of quantum algorithms for problems in financial services. We conclude with a summary of technical challenges and future prospects.
I highly recommend it.
Share this:

This year’s IEEE Quantum Week is planned for October 12-16, 2020, in Denver, Colorado.
IEEE Quantum Week is a multidisciplinary quantum computing venue where attendees will have the unique opportunity to discuss challenges and opportunities with quantum researchers, scientists, engineers, entrepreneurs, developers, students, practitioners, educators, programmers, and newcomers.
The IBM Quantum team is well represented at the conference with a keynote from Jerry Chow. We also have 7 tutorials and 2 workshops.
Our tutorials are on the following topics:
- Quantum programming, an introduction
- Quantum machine learning for data scientists
- Quantum hardware control: a hands-on introduction
- Quantum algorithms for optimization
- Quantum algorithms for chemistry simulation
- Assessing the quality of qubits and quantum computers
- Serious Games for Quantum Computing
Our workshops are:
- Control and design of superconducting qubits
- Software for quantum applications, algorithms, and workflows
Registration is now open.